Séminaire « Optimization and Data Science » à la Sorbonne
Publiée le 2019-04-02
organisé par Ivana Ljubic (ESSEC) et Sonia Vanier (Paris1 Panthéon Sorbonne)
Un grand succès de la journée « Optimization and Data Science » qui s’est tenue à l’université Paris1 Panthéon Sorbonne au sein des sites historiques de l’université, à savoir l’amphithéâtre Richelieu de la Sorbonne et l’appartement Décanal au Panthéon.
Cette rencontre organisée par les deux groupes de travail « POC : Polyèdre et Optimisation Combinatoire » et « OR : Optimisation des Réseaux » rattachés au GDRRO et à la ROADEF, est également soutenue par le laboratoire SAMM de Paris1 et l’ESSEC.
Elle a réuni plus de 110 participants académiques et industriels intéressés par le traitement, l’analyse et la valorisation des données, en particulier par les nouvelles approches basées sur les méthodes d’optimisation pour renforcer les approches classiques en apprentissage et en analyse des données.
Les participants ont pu apprécier cinq excellentes présentations qui ont abordé à la fois les approches de l’optimisation pour l’analyse des données mais aussi les méthodes d’apprentissage pour enrichir les algorithmes en optimisation. Les applications industrielles combinant les deux domaines ont également été présentées.
Quatre imminents chercheurs dans ces thématiques ont présenté leurs travaux durant cette journée :
- Andrea Lodi, Département de mathématiques et de génie industriel, Polytechnique Montréal "On data, optimization and learning" : In this talk, we advocate a tight integration of Machine Learning and Discrete Optimization (among others) to deal with the challenges of decision-making in Data Science. For such an integration I try to answer three questions: 1) what can optimization do for machine learning? 2) what can machine learning do for optimization? 3) which new applications can be solved by the combination of machine learning and optimization?
- Dolores Romero Morales, Copenhagen Business School, Denmark "Learn and Interpret with MINLP" : Data Science aims to develop models that extract knowledge from complex data and represent it to aid Data Driven Decision Making. Mathematical Optimization has played a crucial role across the three main pillars of Data Science, namely Supervised Learning, Unsupervised Learning and Information Visualization. In this presentation, we discuss recent Mixed-Integer NonLinear Programming models that enhance the interpretability of state-of-art supervised learning tools, while preserving their good learning performance.
- Emilio Carrizosa, Universidad de Sevilla, Spain "Cost-sensitive classification and regression" : A critical issue in classification and regression problems is how cost is taken into account. This involves both the measurement cost (and thus, we are interested in having sparse models, since less variables are used) and misclassification/regression errors, which may be of different magnitude and hard to callibrate. In this talk we will discuss a few classification and regression models in which (Mixed Integer) Nonlinear Programming turns out to be a critical tool to address and control such cost-sensitive problems.
- Andrea Lodi, Département de mathématiques et de génie industriel, Polytechnique Montréal "Dealing with uncertainty in tactical planning by machine learning" : In this talk, we propose a methodology to predict descriptions of solutions to discrete stochastic optimization problems in very short computing time. We approximate the solutions based on supervised learning and the training dataset consists of a large number of deterministic problems that have been solved independently (and offline). Uncertainty regarding a subset of the inputs is addressed through sampling and aggregation methods. Our motivating application concerns booking decisions of intermodal containers on doublestack trains. Under perfect information, this is the so-called load planning problem and it can be formulated by means of integer linear programming. However, the formulation cannot be used for the application at hand because of the restricted computational budget and unknown container weights. The results show that standard deep learning algorithms allow to predict descriptions of solutions with high accuracy in very short time (milliseconds or less). A careful comparison with alternative stochastic programming approaches is provided.
- Pablo SAN SEGUNDO, Universidad Politécnica de Madrid, Spain "Advances in combinatorial branch-and-bound techniques for the Maximum Clique Problem" : The Maximum Clique Problem is a fundamental NP-hard problem in graph theory which finds numerous real-life applications, such as pattern-recognition in data science. In the last decade a number of new upper bounds and implementation techniques have come to light, which have improved the performance of prior exact solvers by orders of magnitude. The relevance of these improvements has been such that there has been an upsurge of interest in solving other complex combinatorial problems and applications by reducing them to a maximum clique problem. In this talk, some of the cutting edge improvements concerning exact maximum clique search will be discussed. Special attention will be devoted to the relation of these techniques with other combinatorial problems. At the end of the talk, the topic of pattern-recognition applications concerning cliques will also be addressed.
Plus d'infos : https://www.lamsade.dauphine.fr/~poc/poc/?q=node/59
Speakers and organizers of the workshop on #Optimization and #DataScience at #sorbonne
— Ivana Ljubic (@ILjubic) 27 mars 2019
R. Mahjoub, @pablosansegundo, @emiliocarrizosa, S. Vanier, @ILjubic, @DoloresRomeroM, @69alodi pic.twitter.com/kBmpUu9Z9v
POC and JOR Seminar on Optimization and Data Science - Panthéon-Sorbonne University https://t.co/tLF2SRw5YM #paris @SorbonneParis1 #datascience #Optimization pic.twitter.com/E8OmkhDNXT
— ROADEF (@roadef) 27 mars 2019
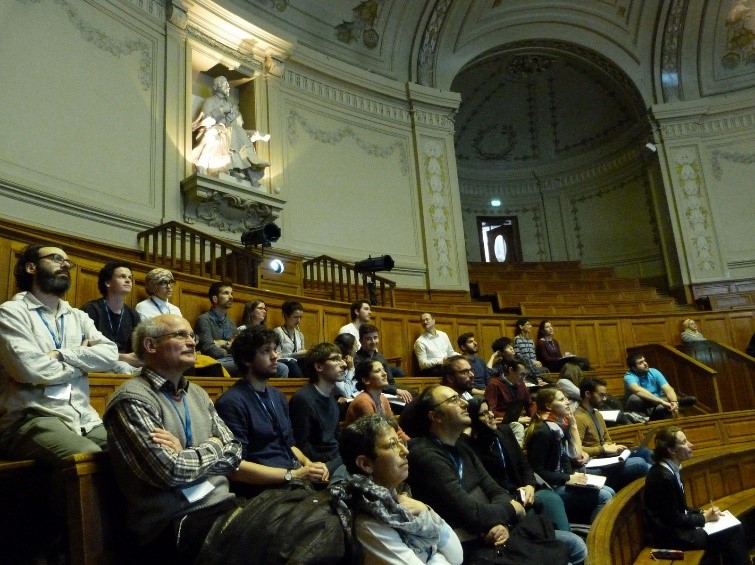